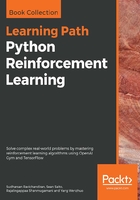
State-action value function (Q function)
A state-action value function is also called the Q function. It specifies how good it is for an agent to perform a particular action in a state with a policy π. The Q function is denoted by Q(s, a). It denotes the value of taking an action in a state following a policy π.
We can define Q function as follows:

This specifies the expected return starting from state s with the action a according to policy π. We can substitute the value of Rt in the Q function from (2) as follows:

The difference between the value function and the Q function is that the value function specifies the goodness of a state, while a Q function specifies the goodness of an action in a state.
Like state value functions, Q functions can be viewed in a table. It is also called a Q table. Let us say we have two states and two actions; our Q table looks like the following:

Thus, the Q table shows the value of all possible state action pairs. So, by looking at this table, we can come to the conclusion that performing action 1 in state 1 and action 2 in state 2 is the better option as it has high value.
Whenever we say value function V(S) or Q function Q( S, a), it actually means the value table and Q table, as shown previously.